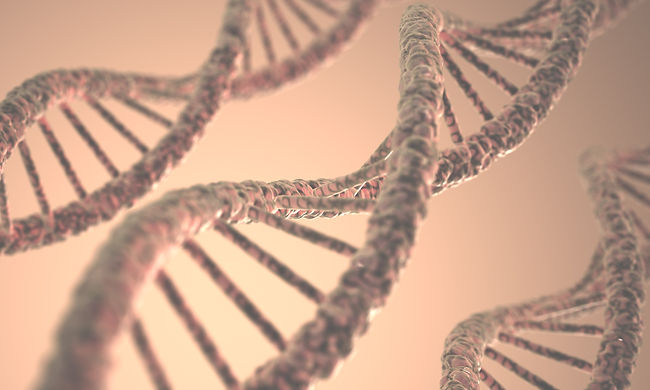
High-performance computing (HPC) started to gain traction in the 1990s with the introduction of massively parallel computer systems. Thanks to steadily increasing compute power and widespread availability of HPC infrastructure, HPC quickly became an essential component in nearly every field of scientific research. HPC is now firmly established as the third pillar of modern research, alongside theory and experimentation.
​
HPC applications can be roughly divided into three classes.
In the first class, modelling and simulation, computer-based models are used as simplified versions of the real-world, reducing the need for costly and time-consuming experiments and allowing the study of physical or chemical properties under conditions that are impossible to test experimentally. Big applications of this class are found in molecular simulation, computational fluid dynamics and climate prediction models, among others.
​
High-performance data analytics or HPDA, the second class, unites HPC with data analytics. It involves parallel processing of large datasets, which may originate from experiments, simulations, or publicly available databases. HDPA is heavily applied in fields such as high-energy particle physics, genomics and proteomics, and optical imaging.
More recently artificial intelligence, and in particular machine learning, is rapidly gaining momentum as a third HPC class. This growing trend is made possible in large part by the wide availability of graphical processing units or GPUs and the development of new algorithms that are able to take advantage of the 1000s of cores on these GPUs.
​
Whenever sufficient amounts of training data are available artificial intelligence can be applied, including bioinformatics, linguistics, medical diagnosis, and astrophysics.
​
​Some research accomplishments on our systems